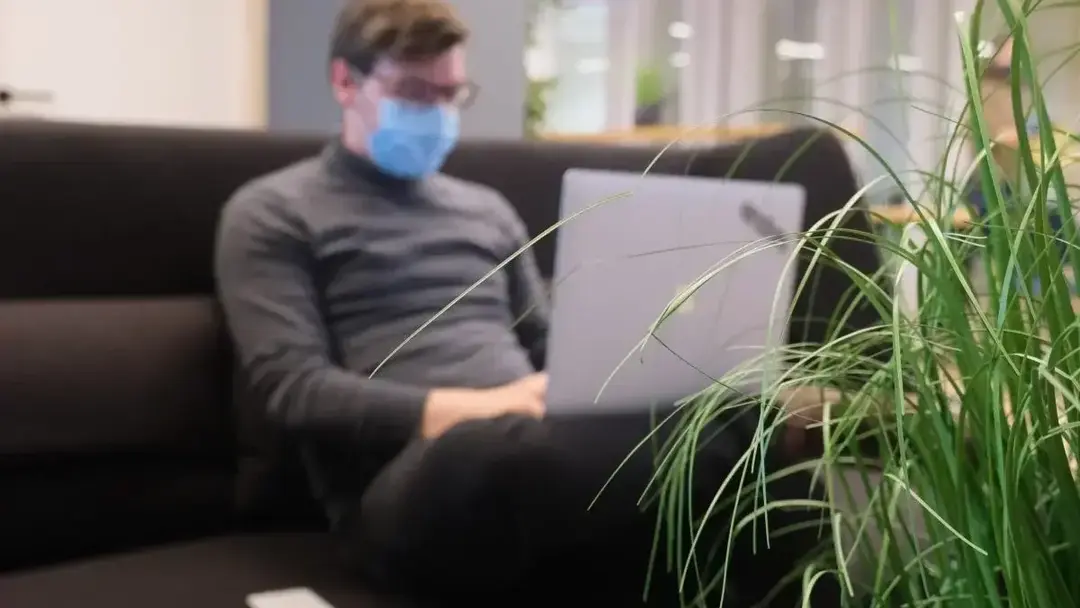
The ripple effect of COVID-19 on machine learning
COVID-19 has disrupted our lives severely in a social, economic and political way. It's hard to predict how the current crisis due to the pandemic will evolve during the coming months or years. Data-driven businesses that have machine learning models in place to gain additional intelligence for customer behavior or sales forecasting, among others, have to deal with this uncertainty and adapt.
The game changed
Many might think that machine learning is not influenced by external factors such as a worldwide pandemic. After all, models are meant to learn from data and adapt to certain circumstances and conditions. While this is true to some extent, many models are directly linked to real-world conditions in such a way that drastic changes in behavior and rules will mess with the outcome.
Major spikes in consumption caused by panic buying and supply hoarding, starring toilet paper and alcohol, lead to demand forecasting models losing it completely. Ahold Delhaize CEO Frans Muller saw the impact and warned the industry for malfunctioning models. Amazon had to implement manual changes to their algorithms in order to keep their warehouses from being overrun.
Recommendation engines kept recommending things that weren't really recommended to do anymore. Think of travel or party-items.
The shift to online shopping for many people caused the amount of detected fraud cases to go through the roof since it was unexpected behavior.
Personalized news sections turned into personal corona-virus dashboards, lacking any notion of sports or cultural news.
Toilet paper and alcohol (gel?) were top priority for people, not for ML models
Mapping reality to machine learning
In short, we responded to the pandemic outbreak in several steps:
First, the exponential progress of the outbreak got detected and picked up by authorities.
Then, early responses involved case detection and intervention planning and lastly a phased implementation and execution of this plan.
This happened step by step and measures were based on certain thresholds in the number of cases, reproduction value and more. The same concept can be used for altering machine learning pipelines.
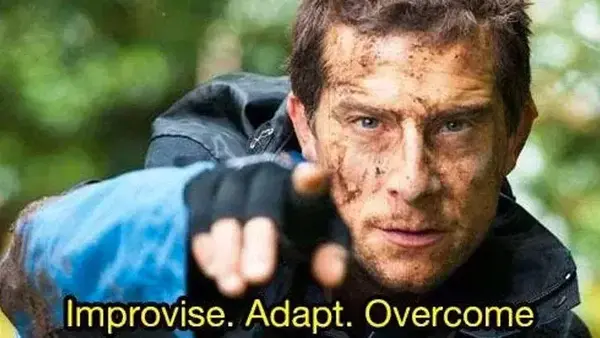
Please fix
To be fair, you can't prepare for everything. If a machine learning model doesn't see what it's expecting to see, problems will show up. Here are a couple of things you should consider:
- Invest in outlier detection
Detecting spikes (demand for a certain product for example) automatically early on is key to intervene. - Perform extensive input monitoring
Building a dashboard that can automatically show you distributions and trends in your input data is extremely valuable. - Take external trends into account
Integrating trends like mobility, news, economic indicators could be useful to trigger certain interventions. - Keep people in the loop
People are still much better at connecting what's going on in the world to what's going on in algorithms and provide context.
Now is the time to ask how we can design these systems better and smarter to be more resilient. We need to watch over and monitor them closely to nurture trustworthiness and reliability!
Interested to know implications today, tomorrow and in the long run?
Head over to our free webinar covering this topic or check out our AI expertise page for more insights and information on designing resilient machine learning systems. Don't miss out on the opportunity to nurture trustworthiness and reliability in your business! Visit our AI expertise page now.
Source: Retail Detail
Book a meeting
Discuss your idea directly with one of our experts. Pick a slot that fits your schedule!